Course of Action Analysis with LLMs: A New Frontier in Defense
The opportunity, challenges, and technical components of LLM-based COAs analysis
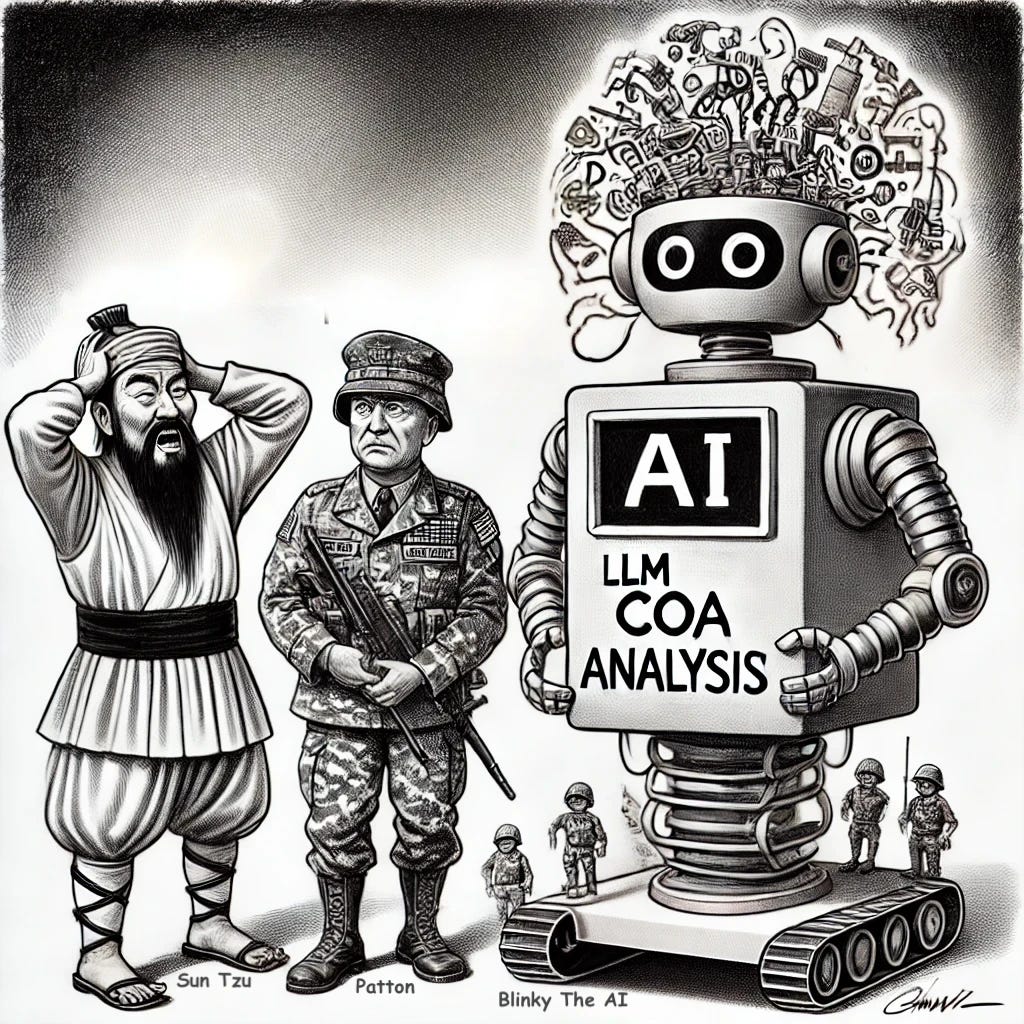
Imagine a world where military commanders can predict enemy movements with uncanny accuracy, adapt strategies in real-time, and coordinate across multiple battlefields simultaneously. This isn't science fiction — it's the promise of AI-powered Course of Action (COA) analysis, and it will reshape how we think about modern warfare.
Recent initiatives within the DOD highlight the pressing need for innovation in this area. The Defense Innovation Unit's Thunderforge project seeks to accelerate and enhance joint planning capabilities while maintaining rigor and human judgment. Simultaneously, an Army SBIR topic explores Boyd's OODA Loop (Observe, Orient, Decide, Act) to discover disruptive courses of action in multi-domain operations (MDO). These efforts aim to empower warfighters by increasing both the rate and rhythm of engagement, enabling commanders to leverage the complexity of MDO for decisive victories through strategic surprise.
The Growing Importance of COA Analysis in Multi-Domain Operations
A grounding document, Joint Publication 5-0 (JP 5-0), titled "Joint Planning," is a key doctrine publication of the United States Department of Defense that provides guidance on the planning of joint military operations. According to Joint Publication 5-0 (JP 5-0), the COA is a critical component of the joint planning process, encapsulating the type of action, its necessity, the responsible entities, and the expected outcomes. Developed during the third step of the Joint Planning Process (JPP), COAs are crafted to achieve mission objectives and must meet specific criteria: adequacy, feasibility, acceptability (balance of cost and risk), distinguishability, and completeness.
As military operations become increasingly complex, the JP 5-0 becomes exceedingly difficult to scale, and the need for rapid and adaptable decision-making increases. Multi-Domain Operations (MDO) require coordination across air, land, maritime, space, and cyber domains. In these settings, COA analysis is a dynamic process, requiring the ability to evaluate various strategies quickly and effectively.
Traditional COA analysis often struggles under the sheer volume and velocity of information, which can lead to delayed responses and missed tactical opportunities. This is where LLMs, coupled with robust data integration capabilities, have the potential to be transformative.
The Evolution of LLMs for Military Applications
As early as the spring of 2023, a few tech companies began promoting their capability to perform order-of-battle assignments using LLMs. However, this was a stretch. At the time, the industry was still in the early stages of working with LLMs effectively. Even the best-in-class approaches were just figuring out how to incorporate Retrieval-Augmented Generation (RAG) workflows, which are essential for connecting LLM outputs to real-world data. Since then, progress has been rapid, with RAG-based workflows now forming a backbone of advanced AI systems in military settings.
Today, the potential for AI in COA analysis has grown further with the onset of advanced reasoning models, tools, functions, and agents. These new capabilities expand the scope of what LLMs can achieve by enabling:
Enhanced Reasoning: Beyond generating responses, reasoning models can engage in complex analytical tasks, considering multiple variables and potential outcomes. This is crucial for COA analysis, where decisions depend on nuanced evaluations of risks, benefits, and operational feasibility.
Tools and Function Integration: By leveraging tools like databases, APIs, and simulation environments, LLMs can now access, interact with, and take actions against a vast array of external data sources in real time. Function-calling capabilities enable these models to dynamically retrieve and process information—such as real-time sensor data or troop movements—providing commanders with COA recommendations that reflect the most current intelligence.
AI Agents for Task Automation: The development of AI agents capable of automating specific tasks has made it possible for LLMs to support multi-step processes in COA analysis. These agents can independently initiate data retrieval, conduct simulations, or assess multiple COAs, freeing human planners to focus on higher-level decision-making and strategic oversight.
By integrating these capabilities, AI systems can now perform more than just static analysis. They can actively participate in dynamic, ongoing planning processes, supporting the DoD’s need for agile, informed, and rapid decision-making.
Addressing Key Challenges
AI systems designed for COA analysis in defense environments face significant challenges, especially in the context of MDO. For LLMs to effectively assist in COA analysis, they must address the following core challenges:
Reducing Hallucinations: In high-stakes environments, inaccurate outputs—often referred to as “hallucinations”—can be detrimental. In COA analysis, the AI-generated plans must reflect reality, as even minor inaccuracies can lead to severe consequences in the field. Current AI models can sometimes generate plausible-sounding but inaccurate information. Therefore, COA-focused AI needs robust systems to cross-reference data and verify the validity of its responses in real time.
Rapidly Updating Knowledge: The battlefield is highly dynamic, with new intelligence emerging constantly. LLMs must be capable of integrating fresh data quickly to maintain situational awareness. In COA analysis, this is particularly challenging as the AI must constantly ingest and process updated information from multiple domains—such as troop movements, environmental changes, or intelligence from various sources—to provide timely and relevant recommendations. This requires a framework that supports near-instantaneous updates and the ability to revise strategies based on the latest inputs.
Integrating Structured and Unstructured Data: COA analysis relies on both structured data (like military logistics or troop positions) and unstructured data (such as news reports, satellite imagery, or open-source intelligence). Combining these data types is essential for a comprehensive view of the operational environment. LLMs must seamlessly bridge the gap between structured and unstructured data, synthesizing information from diverse sources to provide actionable insights. This integration must be rapid and reliable, enabling the AI to handle a wide range of data types that are inherent to MDO.
Optimizing COA Analysis: In traditional COA analysis, human planners spend considerable time comparing multiple strategies to determine the most effective course of action. For an AI system to assist with this, it needs to quickly evaluate various potential actions across multiple dimensions, such as operational effectiveness, risk level, and logistical feasibility. The complexity of MDO adds another layer of difficulty, as it involves coordination across various domains. LLMs can support this process by using optimization algorithms that evaluate and compare different COAs rapidly, presenting commanders with options that are both operationally sound and adaptable to changing conditions.
Operating in Austere and Contested Environments: Modern military operations increasingly occur in environments with limited or contested computing resources, such as edge deployments in the field or situations where infrastructure might be damaged or disconnected. To ensure that commanders maintain the necessary operational control, LLM-powered COA analysis must be able to function in these austere environments. This requires compact and resilient computational infrastructures that can operate with minimal connectivity or support. Edge computing and localized processing are critical to ensure that decision-making tools are always available, enabling field teams to uphold commanders' intent, even when access to centralized resources is restricted.
Other Important Things: Data, Streaming, and Security
Advanced Data Integration and Real-Time Adaptation: A key capability in this approach is handling multimodal data—integrating geospatial data, open-source intelligence (OSINT), and publicly available information (PAI). By leveraging data such as satellite imagery and terrain data alongside textual intelligence reports, AI systems can build a comprehensive picture of the operational environment. This level of integration is vital for MDO, where decision-makers need to synthesize information from diverse sources to make informed choices.
Feedback Loops: Adaptive feedback loops further enhance this capability by enabling real-time interaction between AI-generated COAs and human commanders. This allows strategies to remain adaptable as battlefield conditions change. Commanders can provide feedback to refine COAs on the fly, and the AI system updates its outputs in response to new inputs. This continuous loop of refinement aligns with the OODA (Observe, Orient, Decide, Act) Loop, a key concept in military decision-making, allowing AI systems to provide actionable insights that evolve as quickly as the conditions on the ground.
Secure, Scalable, and Built for the Battlefield: Security and scalability are paramount in military applications. Effective deployment in defense settings requires AI systems to operate in DoD-approved environments, whether in cloud-based or edge-deployed systems. Secure deployments allow for flexibility across various scenarios, from central command centers to austere field environments, enabling reliable COA analysis in all conditions. As capabilities continue to advance, future integrations with other military systems, from logistics platforms to threat analysis tools, are expected to create unified platforms that provide commanders with a comprehensive view of the operational environment. This would help them make faster, more informed decisions and effectively navigate the complexities of modern warfare.
The Road Ahead: Revolutionizing COA Analysis with LLMs
The journey to revolutionize COA analysis within the DoD is just beginning. With the continued integration of advanced LLMs, adaptive feedback systems, and real-time data inputs, AI platforms are positioned to deliver faster, more accurate, and adaptable decision-making capabilities. These advancements will set new benchmarks for speed, accuracy, and adaptability in COA analysis.
By bringing these technologies to bear on the challenges of MDO, the DoD is paving the way for the future of defense. Equipping commanders with AI tools designed to meet the demands of complex, multi-domain battlefields ensures they can respond with confidence, agility, and precision in the face of evolving threats. This is the future of military decision-making—and it’s within reach.