Author’s Note: This three-part series was sketched out over the spiked eggnog while watching my favorite Christmas movie: Star Wars. Wut? The focus is on the challenges GPT-4 will face before it really becomes a true driver of economic growth for enterprises.
Staying true to the series, I begin with Episodes 4-6, “GPT-4 is the Death Star,” followed by Episodes 1-3, “The Rise of EmpireAI,” and closes out with Episodes 7-9, “He’s Going Full Palpatine.”
Thank you to Sam Lau for yet another awesome cartoon!
GPT-4 is the Death Star. We don’t yet know the full strength or size, but rumors place it well beyond the recently released and very impressive ChatGPT. We know millions of users are providing feedback on OpenAI’s platform. And we know OpenAI has a lot of room to run with $10B in funding from MSFT. So it seems fair to expect in 2023, GPT-4 will arrive as a fully armed and operational battle station coming to blow up life as we know it.
However, like any good Death Star, you can rest assured, this Death Star was designed from the beginning with structural flaws, and yes, it will be destroyed. Why? If GPT-4 has similar characteristics to its predecessors (ChatGPT), many users and most enterprises will find that they:
will not trust the non-factual results and will constantly have to edit and double-check everything written;
will not be able to use the GPT-4 because it has no knowledge of current events/trends;
will get frustrated by consistently adding written context related to their jobs, relationships, and organization;
won’t want to share all of their sensitive corporate information with OpenAI/MSFT; and
will struggle to justify the cost of the service (for all the reasons above).
So, let’s dive into this Trench Run and go through the hype, the delusions, and the design flaws of the Imperial AI superweapon.
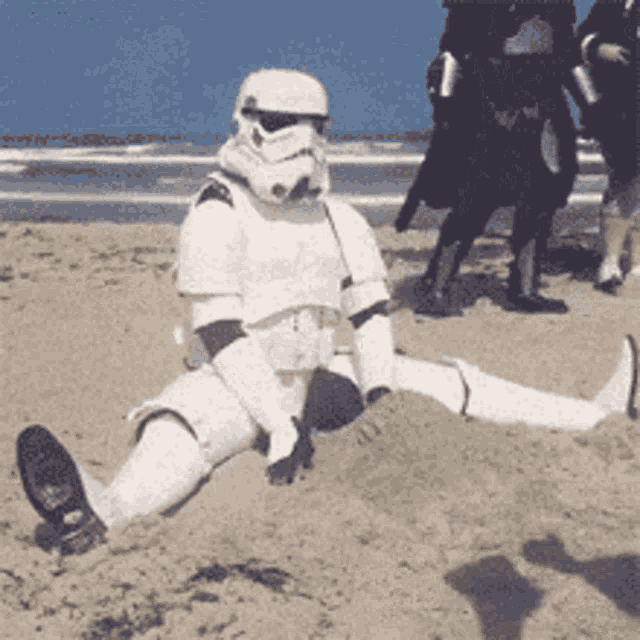
The Hype
The accolades, expectations, and fears of industry disruption are all over the media and The Twitter. Several outlets speculate that OpenAI/Microsoft (OAI/MS) and ChatGPT may kill the Googliath of Search. Given Google’s search business is 10-15 times the size of Microsoft, there’s room for OAI/MS to gain ground, and GPT-4 will be at the center of the strategy (but let’s not make ‘Bing it’ a thing).
In another example, GPT-4 appears to have its sights on planet Alderaan aka the entire education industry. Turns out ChatGPT is pretty good at writing essays, GPT-4 will be better. Bans, Honor Codes, and detection tools may discourage GPT-4 in the short term, but in the long run, it’s fair to believe GPT-4 will change how we learn and may actually make us better writers.
In a final example, GPT-4 will likely build on ChatGPT and place newsrooms across the globe on notice. CNET recently paused its AI publishing practices over concerns about journalistic standards. ChatGPT has placed a spotlight on the process of automation of news with the speed at which relatively quality output can be produced. But in an era of squeezed margins, will journalists become beholden to GPT-4? Stand together or die together.
The Delusions
The most Death Star-like press and predictions revolve around the GPT-4’s automation and replacement white collar jobs and total shock on the 2023 economy. That feels a bit ridiculous in the near term, not only because of the limitations of the technology, but also how work actually gets done. To deeply influence the economic output of most companies and industries, GPT-4 will need to penetrate and integrate within enterprise IT infrastructure, procedures, and training. That may take years to a decade or two to accomplish.
How will MSFT pull off such a massive transformation? “Pulling a Teams” aka, integration and distribution of ChatGPT (and likely portions of GPT-4) into MSFT products and services. Full integration into massively used productivity tools does more damage than a lightsaber in Mos Eisley Cantina, but widespread distribution of massive models will be partially dependent on using MSFTs cloud platform. Given that MSFT only controls roughly 21% of the global cloud market in 2022, full use of GPT-4 will be partially market limited, and we won’t be a tectonic shift away from AWS and Google, which means we will unlikely see a GPT-4 total economic shock in the next few years. Over the next ten years? Impossible to see, the future is. The keys to real enterprise adoption and economic impact will be determined by how fast OAI/MS addresses a series of design flaws.
The Design Flaws
For all the hysteria surrounding OpenAI and GPT-4, we need to remember that the Death Star was blown up…twice!….First from a 90° bank shot in a womp rat-sized hole, and the second by Billy Dee “works every time” Williams.
When it is released, GPT-4 will seem to be an inevitable force to take over society, but to truly impact industries and the global economy, five hurdles will stand in the way:
factual outputs for writers and enterprise users;
the recency of outputs to capture current trends, events, and language;
contextual corporate knowledge required to be actually useful;
the cost profile for hosted, private cloud, and on-premise/edge; and,
the ability to address privacy and security concerns.
Flaw 1: Truth ...” It’s a trap!” The Mon Calamari Admiral found out that the Death Star II was fully operational when it was said to be under construction. Akbar lived to see another day but provides a good lesson not to rush into something too good to be true.
ChatGPT is mind-blowing at first glance. It feels almost limitless as a writing assistant and provides a visceral experience for some. After speaking with dozens of users, the strongest initial feeling is associated with, the “overcoming the blank page” problem. So much time is spent trying to kickstart a memo or paper, but when people try ChatGPT, they feel that it could easily get them over the hump. Understanding that feeling is important. It highlights a massive pain point addressed not only by LLMs but by the ingenious experience that ChatGPT provides as a basic chat assistant. Kudos to OpenAI.
The incredible initial experience almost justifies the accolades calling it the equivalent of the calculator. However, it is most certainly not yet a reliable writing assistant, because so much of what it writes is not certain. Rather, as Ezra Klein eloquently states, “It is b*llsh*t….it is content that has no real relationship to the truth.”
This is where the largest problem exists for OAI/MS. Consistent use of ChatGPT requires a very different engagement pattern. Every sentence that contains real information needs to be reviewed, every fact must be checked. The time savings that appear to be instantly realized by using ChatGPT, quickly disappear. Users may spend more time tinkering with prompting and fact-checking, than just sitting down and writing. As perceived gains turn into diminished value, ChatGPT may see real churn in the monthly subscription business model, and will quickly erode trust in other LLMs.
Will GPT-4 save the day? GPT-4 will likely be even more convincing and accurate with its output, but what remains to be seen is now it will address actual facts. There are a few published techniques on how to link facts (and knowledge graphs) to generative text, and I’m sure OAI/MS has some non-published approaches. I believe it will address facts/claims by connecting to Microsoft’s Bing Search engine to calibrate and go after Google’s Search business. If OAI/MS doesn’t address the lack of factual output for most written tasks, or it punts that feature to ChatGPT-2, GPT-4 will feel at best incrementally better than ChatGPT and will be questionably useful and potentially risky for enterprises to broadly roll out.
Flaw 2: Recency. The original plans for the first Death Star were drafted around 25 years before it went online – following the storyline, that’s Attack of the Clones time. Apparently, touch screens were not yet a thing.
ChatGPT is many things, up-to-date on current events, but it is not. The information is current as of 2021. It knows nothing about Russia’s invasion of Ukraine, Elon didn’t own Twitter, and the stock market was peaking. Technologies change, competition changes, and the language for many industries evolves quickly.
The recency gap is an interesting miss as ChatGPT’s best current use case – is the mass production of SEO blogs. Unclear where GPT-4 goes on this front. Adding incremental information is a relatively solved problem, but it comes at a cost, through some forms of retraining/augmenting the model. However, OAI/MS chooses to solve it, I’m guessing ChatGPT user logs are showing it’s an important flaw to address.
Flaw 3: Context. The Holiday Special has nothing to do with the Death Star, but it’s the best example I could think of and the Holiday Special is a necessary reference.
ChatGPT shows an extensive breadth of understanding and depth in many areas, yet it struggles to show depth at both an industry level and within organizations. GPT-3 is an even worse experience. Some rumors say that OAI/MS is taking this head-on and training GPT-4 at a similar size to GPT-3, but with a much more expansive data set. This would likely add depth to the overall experience but will miss organizational language, styles, and relevant information.
Which begs the question: will OAI/MS rely on third-party applications plugging in GPT-4 their corporate information (Wikis, Notion, Slack, CRMs, CMSs), or will they build native plugins to key systems of records themselves? Companies like Jasper were built on GPT-3 from the beginning Notion plugged GPT-3 into its application recently, and an entire Trade Federation of startups is raising venture capital to enable OAI/MS. These are all positive steps, but by themselves, they won’t unlock game-changing economic value for companies. The true gains will be when LLMs like GPT-4 will look across systems of record, and provide contextual information at a user level.
Flaw 4: Privacy. One great thing the Death Star had going for it was a state-of-the-art tractor beam. It was wonderful at locking in ships, pulling them in, and not letting them back out.
GPT-3 was a one-way exchange. Your data goes in, and you can fine-tune a model, but that model and weights stay with OpenAI. This approach, also followed by most API competitors, prevented companies from downloading the new model and gaining all of the benefits of the GPT-3 architecture.
ChatGPT provides a chat interface and has been free to date. This has given OAI/MS incredible user feedback in terms of how people may want to use this chat interface, what topics are most interesting, and where are there gaps.
A recent Time survey showed that 30% of their respondents have tried ChatGPT at work. This is a fairly incredible number given the service is only two months old. It also should raise alarm bells for CEOs, CISOs, and Corporate Counsels. As companies rely more and more on engaging with an API platform like ChatGPT or GPT-4, they’re inherently sharing an incredible amount of corporate knowledge with OAI/MS, and that could come back to bite them.
A massive security breach of this system would be like LastPass on steroids, as it could include extensive corporate information across industries and within organizations.
Cybercrime aside, an even more interesting issue is to consider the potential ramifications of OAI/MS using prompt/query information for their own corporate decision-making. The closest comparison is Amazon using market information to build competitor products under the Amazon Basics brand. Clearly, Amazon had asymmetric information, and it lead to multiple lawsuits and one insanely funny video created by my good friend Pete Dering.
Let’s assume OAI/MS gets corporate security right and they somehow don’t stick their hands in the cookie jar of interesting corporate data, there is one last issue. Companies will not want their data used to train future models as it could benefit (directly or indirectly) their competitors.
So where will GPT-4 take privacy? Data will be a potential moat for future higher-quality models, but corporations are going to be excited to send it over to OAI/MS cloud. Sharing for future training of models is scary for most, and well cybersecurity seems to continue to be a burden. So, will GPT-4 deploy directly to company servers? Ooof that may prove costly….
Flaw 5: Cost. Estimated to cost between thirteen thousand to two million times Earth’s GDP, the Death Star was an absolute beast to finance and build. The cost to build GPT-4? Estimates range from $100M - $2.3B, so no one knows. The potential model size (parameters, data) is debated more than Mark Wahlberg’s reveal in Boogie Nights.
Recouping the training costs can be done to some extent by spreading over many customers and industries. However, inference costs may be a real issue for many enterprise customers. An instance of GPT-3 (175B params) requires 700GB of GPU memory, which translates to roughly 50 GPUs (16GB memory) simultaneously for every inference. What if GPT-4 is 2T parameters?
If OAI/MS is hosting GPT-4 instances, it can share the inference costs across many individual customers, but how many companies will want to pay for a privately hosted instance of GPT-4? Dozens or maybe a few hundred? Moreover, what will happen when enterprise customers want to host their own private version (like every bank, the DOD, and other regulated industries)? It’s going to be a non-starter.
The end of GPT-4. Here’s the rub, it is highly likely that with the way OAI/MS will roll out GPT-4 to enterprises, there will be a tradeoff. If OAI/MS doesn’t design GPT-4 in a way that represents facts/claims, recency of information, or organizational context, the usefulness of GPT-4 will be degraded. If it doesn’t address privacy and security concerns, using GPT-4 will be a non-starter for many enterprises, and if it does address all of these issues with a very large architecture (>GPT-3), the cost profile of the service will likely not justify widespread enterprise adoption, and it won’t unlock the macroeconomic gains.
So, are these the devastating design flaws that will doom the GPT-4 Death Star? Yes and no. If GPT-4 has these issues, the hype will be great, but real adoption in the enterprise will be slow. What will kill GPT-4, which will be covered in, “He’s going Full Palpatine.”
Disclaimer, my perspective on GPT-4 and OpenAI is factual but biased. At Yurts AI, we’re working on ways to bring LLMs into enterprises and how to fix major drawbacks. Kudos to Sam & the Open AI team, they have brought LLMs and Foundational Models (FMs) into the cultural discourse faster than the Millennium Falcon made the Kessel Run.
Well-done BVR.
> Trustworthiness of results: While it is true that AI models like GPT-4 may not always provide 100% accurate results, it is important to note that they are designed to generate human-like responses based on the input data they have been trained on. The results can be improved by fine-tuning the models on specific domains, providing them with more relevant training data, and using proper evaluation methods. It is also the responsibility of the user to verify the results before using them, as with any other tool or technology.
> Lack of current knowledge: GPT-4 is trained on vast amounts of text data from the internet, which includes current events and trends. However, it is important to note that AI models do not have the ability to automatically update their knowledge in real-time. This is why it is necessary to regularly fine-tune the models with the latest information.
> Frustration with context: Providing additional context to AI models can help improve their results, but it is important to note that this is a common issue with many AI technologies and is not unique to GPT-4. With proper training and fine-tuning, the model can be improved to better understand the context in which it is being used.
> Concerns about sensitive information: It is understandable that some organizations may have concerns about sharing sensitive information with third-party providers like OpenAI and Microsoft. However, it is important to note that reputable companies have robust security measures in place to protect their clients' data. Additionally, many companies offer privacy options and allow users to control the data they share with the service.
> Cost justification: While the cost of AI services like GPT-4 may seem high, it is important to consider the benefits they provide in terms of productivity, efficiency, and accuracy. These services can help organizations save time and money by automating repetitive tasks, freeing up employees to focus on higher-level work. Additionally, the cost can be justified by the increased accuracy and efficiency of the results provided by the AI model.
In conclusion, while AI models like GPT-4 have the potential to revolutionize the way we work and interact with technology, it is important to understand their limitations and limitations. Rather than viewing AI as a magic solution to all of our problems, we should approach it with realistic expectations and understand its role as a tool to augment human intelligence rather than replace it.